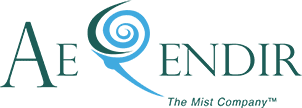
FindBiometrics President Peter O’Neill recently had the opportunity to interview Martin Zizi, CEO of Aerendir, a leading pioneer of novel physiological biometric identity technology.
Their conversation begins on the topic of what physiological biometrics are and how they differ from traditional modalities like fingerprint or face recognition. Zizi goes on to discuss why it’s important for the industry to focus on implementation rather than comparing modalities, and expands on the privacy-enchaining aspects of Aerendir’s technology. The interview concludes with Zizi and O’Neill delving into the fascinating use cases for physiological biometrics – including how Aerendir’s technology can solve the bot amplification problems on social media and across the wider internet – and the importance of proof of work and knowledge in the era of blockchain.
Read the full FindBiometrics interview with Martin Zizi, CEO, Aerendir:
Peter O’Neill, President and Founder, FindBiometrics: What are physiological biometrics and how do they differ from other biometric modalities?
Martin Zizi, CEO, Aerendir: Physiology means they are based on vital signs whose signal is always different, never the same, and involuntary. We can think of it in the way the heart beats, and in the way it represents you. The voice is also a vital sign that constitutes a form of physiological biometrics. Neural activity, which our company specializes in, is another example. If you look at the iris, some neural activity is reflected in the micro-vibration at the center of the eye.
The core qualities of physiological biometrics are that they have to have vital signs, functional activity with a signal that is never twice the same, and is involuntary. They differ from other biometric modalities because they aren’t anatomical features. The best example is the fingerprint: it is part of the anatomy, it doesn’t change, it isn’t functional, it doesn’t evolve, and it is like a picture.
Behavioral biometrics like walking or the act of doing a specific motion like waving a hand or signing a signature differ from physiology because they are contact-dependent and voluntary.
Peter O’Neill, President and Founder, FindBiometrics: You know, what I find very interesting about this, Martin, is that one of the big topics in our industry right now is liveness. It would appear that your solution has it built in. Is that correct?
Martin Zizi, CEO, Aerendir: Yes, but it isn’t only my solution. If you were to analyze heartbeat, voice, breathing and even the iris micro motion – they all have built-in liveness capabilities which solve quite a few problems in our field, as you know. As an example, biometric systems built with liveness detection can prevent spoofing attacks.
Peter O’Neill, President and Founder, FindBiometrics: Why do we need to focus on how biometrics are implemented instead of what modalities are used? Could you explain that for us please?
Martin Zizi, CEO, Aerendir: Yes, with pleasure. I’ve been around a few years now and I see that the public, the stakeholders, the businesses, the big clients or different use cases are misled, not intentionally but because we focus on the wrong thing. It is like a competition where my biometrics is better than yours, I have a better recognition rate, I have a better failure rate, and it is all good because you need performance. But the problem is we have to look at the law of unintended consequences, and I will walk you through three examples that are prevalent for people, because not everything is safe.
For example, having a database: we have our names in databases, credit cards in databases, social security numbers – and we may lose this information in the case of a breach. We will survive even if we lose a few thousand dollars, but if we have a database that has a large scale of biometrics whether it is a fingerprint, or a voice or even a neural print whatever, and there is a breach, you can’t get a new set of fingerprints, you cannot get a new face, you cannot get a new voice etc. So you are lost in the system forever. So, you can use a biometric x, y, or z, but beside the performance by design you shouldn’t store it in a database because that will be unsafe and will go against the initial need.
Another example, is essentially beaming info. When we use biometrics, and all biometrics can do this, we beam the identity across the IoT, between the phone or mobile device and server. That is not the smartest thing to do because the info can be captured and essentially I am sending to the planet my personal information and that is not smart. The alternative to that is tokenization, but you need to protect the token otherwise you might misplace it, or it will get stolen. There are protocols and technology to do that like the FIDO protocol which will become a standard. So, essentially don’t beam things.
A third example is the fragility. Most biometrics these days use AI but 80-90 percent of it is based on neural nets alone. That is the best and the worst thing because a neural net is like a cathedral; you can, with a lot of data, recognize patterns that you wouldn’t see by human analysis, you don’t know what it is but it is recognized. But the neural net does a lot of things in the linear, and like a cathedral, if you were to remove a few stones from a notch, the whole cathedral collapses. This is what we have seen recently in tech data and in data from research labs that can crush the efficacy of neural nets to zero. So, if you have a biometric database on neural nets alone, well, then good luck because you might be hacked or destroyed very quickly. My suggestion is don’t put all of your eggs in a single technology – you want to use neural nets and other classifiers. When you mix different ingredients you not only make a tastier dish but it is more resilient, if I may use the cooking comparison. Because at the end of the day, if you put all your eggs in one technological basket you might end up being very fragile.
And I come back to the ‘How’ versus the ‘What’ – the ‘What’ is easy, it is testing, and you can combine biometric A with biometric B to get the level of performance required for the specific use case. But the ‘How’ is more relevant because performance is not the only metric. It has to bring safety to people, it has to have resilience against hackability – that was my example referencing the database: it has to be resistant to spoofability. Imagine all the imaging systems we use with biometrics. Hollywood, with the computer graphic imaging, has solved a lot of that and can fake it very well. Did you know that James Dean will be in a movie even though he died in the sixties? People will be spoofed. So, resistance to spoofability is essential.
Most people who use image-based biometrics string it by statistics, that is what people do with big data and neural nets. But learning by statistical analysis remains brittle, meaning you can have a fake profile opening 20% of all the user profiles generically and there are ways to do AI not learning by statistics but learning by failure like the brain does. We do AI by learning from mistakes. If you do that you are less brittle, you are less subjected to being spoofed or breached. So, I think it is fine that the community of stakeholders gets to see the full picture – performance, resilience, hackability, spoofability and once you have these metrics, I think you can make a better choice for the public or for the business depending on the site and requirements.
Peter O’Neill, President and Founder, FindBiometrics: You touched a little bit on the next question which is with all of these data breaches going on, privacy is such a critical component. Can you elaborate a little bit more on your solution and how it enhances privacy?
Martin Zizi, CEO, Aerendir: I thank you for the question because that is a subject very near and dear to my heart. I think in a shared economy and in a system that will become prevalent in our lives, as a user and a citizen, I have to be in control of my privacy and my personal data.
What we do at Aerendir with our live neurophysiologic biometrics, is we don’t use a database at all. Everything is done on the device; nothing is sent to the Cloud for computation and nothing is beamed. Essentially you train a device, it can be a phone or a tablet, it takes let’s say five minutes of data to train and the five minutes of data cannot be done in a single time, it has to happen at various times of the day and it happens continuously in the background when you use your phone. You and I touch our phone for about 80-100 seconds, and this is ample time for the phone to be touched, to collect some data, and to learn. Once the learning is done every computation on the device is in a few minutes, and again we have an AI where everything resides on the device but learns from mistakes. It is a very complex process but to cut it short we don’t do statistical analysis, we do refined mathematics at a high level and once the model is completed, which takes five or six minutes to do, then the power of the lock is unleashed and you can either use it or not as you decide. So, no database, everything is on the phone at all times.
The locality of the AI, I think, is not only an important feature for biometry, for my company, but also for the future of IoT. If you don’t use local AI you will have latencies and other problems. 5G will not solve it all, it will improve it but it won’t solve it. So, there is a need for local AI at the edge, or I like to say: at the edge of the edge.
Then we are actually a dual factor. I combine a phone and a hand. Why I say a single phone – because no two phones are alike. Their motherboards have different failures, different notes so, there is an electronic idiosyncrasy for phone A which is different for phone B that I fuse with the electronic pattern that I can take from the nano or micro motion from who you are and I fuse them both. So, essentially, I have a dual factor because it is a phone and a hand which makes it very solid. If you lose your phone you get a new one and no one can enter yours. Even if someone could try to capture your physiological data and try to play it back, they can’t unless they have your own phone, because 50 percent of the system is missing.
This is quite strong; you have a frictionless dual factor free of charge.
The way we do the mathematics, as I told you, is: we train the AI not by statistics but by learning from mistakes, and this makes it immutable and unspoofable. Along the very refined mathematical equation you don’t know how to generate a fixed signal until you know all of the equations. By just talking about statistical analysis of micro-motion or brain activation of neuro pattern you will fail because the signal will never pass for the real signal of Mrs. A or Mr. B, because it is more refined than that – it is not a direct statistical method.
For all these factors, we offer efficacy and safety but at the same time we protect privacy. I can say your modelization of your neural pathway is akin to a one million character long password and we are busy building super encryption. At the same time I can say that the future of your data is in your hands, your identity is you, your body is you, but you control it because it always remains on your device. And at the same time, your brain can also shield you from prying eyes because of the encryption functions.
Peter O’Neill, President and Founder, FindBiometrics: Can we talk a little bit about actual use cases for your technology? Can you give us some examples where it is being used right now, what vertical markets, that kind of thing?
Martin Zizi, CEO, Aerendir: Yes, of course. First, before I get into the four different use cases, I want to mention that we can be embedded at various levels in various products and ecosystems.
We can function 1.) as an app, 2.) we can be part of a firmware or an operating system, and because of the way we design our code, the way we code, and the language we use, 3.) we can be ported to microchips like the application-specific integrated circuit which is ASIC.
The first tier of use cases is authentication. The process for that is in association with phone carriers because the phone can be used as a conduit to payments and service and there is need for strong payment authentication. I am not a bank and I need a big friend to go to the market in a B2B environment, and I provide a revolutionary technology, you provide the consumer base, and everyone is happy.
Then, recognizing you from me is a challenge that I have solved, and also recognizing you and me from a bot, a non-human is easy, and we have done that. So, the second use case that we have field tested is a solution for the bot amplification problems that we have seen on social media, and at the same time to clean up the internet bandwidth. People may not know but 50 percent of internet traffic is not human generated. It might be beneficial for businesses, but it drives us all down in terms of resources, social media traffic, the price of advertisements which does not reflect reality. So, in the end, there is a need for a fool proof bot vs. human criteria. When you submit a post, let’s say to a social media platform, at the same time in less than a second, it feels and analyzes the human shiver and it will send a green or red flag on whether this was human generated or not. This is effective to 94 percent, so in a way this would solve the acute problem of bot-generated traffic.
A third use case is that we can measure data that was inaccessible even a year ago. When you open a door between the brain and the computer you can determine gender and age with a high level of efficacy without having to know that you are Peter O’Neill or I am Martin Zizi. So, essentially it could lead to ethical metadata collection instead of breaching any identity without the person knowing that the collection was taking place, because gender and age is reflected in the nervous system.
These are the three use cases that we are busy pursuing business-wise with various partners.
I told you how we can be on the chip and recently we made a deal with a chip design platform called SiFive – which we announced in the press – to make and generate ultra-cheap AI for the case at ‘the edge of the edge.’ This is a technology case which means I don’t build the product but I will help engineer the chip for others to buy and put into their own products, whether it is put in a coffee machine or a stop sign on the road, it is not in my hands.
The fourth level of use which could be years from now – but maybe not depending on how successful we are – is the blockchain. The blockchain, for me at least, is the future of the internet because of the stability of the block which could stabilize and decrease friction in a lot of sections of the economy. Combining a strong authentication, which is what we offer, with the blockchain is a win-win. The blockchain will find a huge level of application and we will provide an essential in-and-out which is lacking at the present time.
Peter O’Neill, President and Founder, FindBiometrics: One final question: how proof of work and proof of knowledge improve biometric systems in general across the board?
Martin Zizi, CEO, Aerendir: I just told you that blockchain has a huge future, but it has various problems to solve: first is the in-out, which I just spoke about. Then: should the data across the blockchain be stored or encrypted? That is the question. Everyone knows that cryptocurrency has to be mined and that there has to be proof of work, which means you have to make it as rare as gold. That is the process of mining, and this proof of work is simply an immense amount of computation that can create a coin after a gazillion of bit operations. Then we call that proof of work, and it requires an immense need of electricity, and people complain about that.
What is proof of knowledge? An example might help: Let’s assume you want to buy a car and you want a price for the car loan and you have a certain set of criteria. The dealership needs to know are you getting enough money with the loan and you need to go through credit checks etc. but at the end of the day you need to give proof of your salary – they need to know your credit rating, etc. This might be personal information that you might not want to share with just anyone. So, with proof of knowledge any information like this can be put into a formula as a precaution. Let’s say to acquire the loan you need to make $200,000 per year and you know that. The proof stays on your device, scans it, analyses it and then makes a formula which will give a result, for example, 50. 50 is an arbitrary number because the proof that is requested becomes part of an equation that converts the data into metadata and this is proof of knowledge. If it is done safely and securely this is the only information that you give to the blockchain to secure your car loan, because above 50 means you are okay, it is like a green flag. Below 50 means you might need a special deal, and below 20 means you don’t qualify.
There are many, many examples of where you don’t want to divulge the data or the knowledge that you have but you can safely share metaknowledge thanks to mathematics. In an open environment that would not be encrypted like the blockchain then there is no risk to put out your personal information like your name, address, financial, directly readable on the block. As of today, I’m not sure if the blockchain will be fully encrypted or not, the debate hasn’t been settled on that. That is where proof of knowledge might be beneficial.
Peter O’Neill, President and Founder, FindBiometrics: Thank you so much for describing this for our readers. I was a pleasure to speak with you today.
Martin Zizi, CEO, Aerendir: Thank you for having me, I appreciate it.
Follow Us